THE INTERSECTION OF ARTIFICIAL INTELLIGENCE AND NEUROSCIENCE
The Intersection of Artificial Intelligence and Neuroscience
Anna Carpio
Clinical Research Associate, Clinical Research Strategies
May 2024
The Introduction and Development of Artificial Intelligence
The Food and Drug Administration (FDA) defines artificial intelligence (AI) broadly as the development of intelligent machines and computer programs. One technique of AI is machine learning (ML) in which developers train software algorithms to learn from data and data sets.(1) In 1964, Corwin Hansch pioneered the first computer-assisted identification method of biological activity and chemical structure, more commonly known as quantitative structure-activity relationship (QSAR), used in the development of pharmaceuticals.(2) As computer programs continued to develop, the first AI/ML-enabled medical device approved by the FDA in 1995 was the PAPNET testing system that used an automated computer system to read cervical cytological slides. Following PAPNET’s initial approval, there were only a handful of AI/ML-enabled medical devices that were approved every year, until 2016, when 18 were approved. A steady increase of approved AI/ML-enabled medical devices has been seen after 2016, as depicted in Figure 1. The rapid increase of approvals may be due to the continuous advancement of computer programs, greater accessibility to larger data sets, and an increase in companies funding AI/ML platforms.(3) In 2023, the estimated global market for AI was valued at $196.63 billion USD.(4) The AI market is predicted to continuously expand by 37.3% annually from 2023 to 2030 based on adoption of AI in various industries, including automobile, finance, and healthcare.
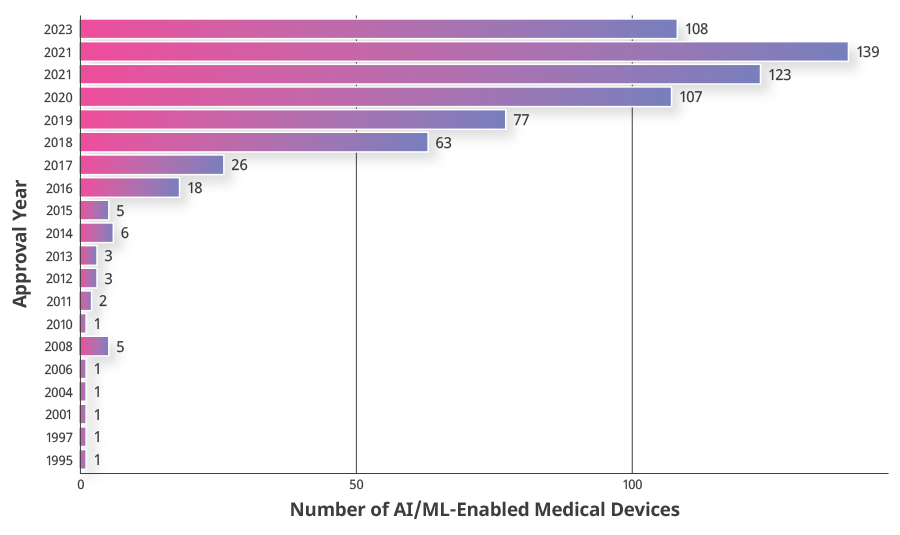
Figure 1: The Introduction and Development of Artificial Intelligence Annual trends in the FDA approval of AI/ML-enabled medical devices from 1995 through 2023.
A more recent discipline of healthcare that has adopted AI/ML is neurology, which includes developments such as neuroimaging, predictive and current diagnosis/treatment, and drug development. As of October 2023, there had been 20 AI/ML-enabled neurological devices approved by the FDA.(5) BrainScope developed the first neurological AI/ML-enabled medical device approved by the FDA in 2014.(6) The device is an AI/ML-enabled device marketed to assist healthcare providers in the diagnosis of concussions or mild traumatic brain injuries (TBI). The device’s main function uses a patient’s electroencephalogram (EEG) data as a biomarker and point of measure. The ML algorithm determines the likelihood for the patient to have a structural brain injury and classifies the severity of the TBI using a baseline EEG database, patient demographics and symptoms, and neurocognitive assessments. The technology is intended to be used alongside other diagnostic tools but aims to reduce unnecessary CT scans for less severe TBIs.(7) BrainScope has undergone several iterations to further develop their technology and is a supporting example of the necessity of the evolution of FDA landscape regarding AI/ML-enabled medical devices.
Due to an influx of AI/ML-enabled devices in neurology and other therapeutic areas as well as AI’s involvement in pharmaceutical development, the FDA has published discussion papers and guidance documents related to the usage of AI/ML. Using the FDA guidelines as current regulatory thinking of the agency, this case study evaluates the efficiency of medical device pathways in relation to AI/ML and assesses the potential impact of AI/ML-based pharmaceutical developments for patients.
Regulatory Pathways for AI/ML Devices
In the discussion paper published on April 2, 2019, the FDA discusses how AI/ML-enabled devices are currently regulated and proposes principles for a future regulatory framework.(8) What may have prompted the push for changes in regulations is the concept of “unlocked” algorithms within device software that updates using real-world data. As of 2022, there are no AI/ML-enabled medical devices with an “unlocked” algorithm that have been approved by the FDA.(9) However, the FDA has approved AI/ML-enabled medical devices that have “locked” algorithms which are restricted to the data set that developers originally used to train the algorithm. The AI/ML-enabled devices that have “locked” algorithms must undergo additional FDA premarket reviews to include any software changes, if not already outlined within the original submission.
The current pathway for submitting a software change to an existing medical device is through subsequent and sequential 510(k) submissions as these changes are based on performance, inputs, and intended use. The purpose of the submissions is to allow the FDA to ensure the safety and efficacy of the software change since the change may affect the original intended use of the device. BrainScope’s regulatory history provides an example of this regulatory pathway.
BrainScope pioneered the first submission of a neurological AI/ML-enabled medical device to the FDA, and since the first submission, they have had six 510(k) submissions that continuously build off their original De Novo submission. These submissions change the algorithm’s inputs which include comparisons of neurocognitive performance tests that result in novel data categories, the creation of a new classification of structural head injury using electrical brain activity data, and the adjustment of the criteria of inputted data based on the patient’s age, time frame of injury, and their concussion symptoms. BrainScope’s submissions spanned over a period of five years with the most recent submission occurring in 2019.(10-13)
The need for subsequent and sequential 510(k) submissions does not facilitate post-market software changes easily. The potential for “unlocked” algorithms, as well as the numerous submissions for “locked” algorithm changes, poses a need for a Total Product Life Cycle (TPLC) approach to the regulation of AI/ML-enabled medical devices with the aim of allowing adaptation and improvement from real-world use of the device. In the discussion paper, the FDA introduces four general principles that the proposed approach follows:
- Establishing standards of quality systems and good machine learning practices (GMLP);
- Conducting premarket reviews for Software as a Medical Device (SaMD) to ensure the device’s TPLC is safe and effective throughout all stages of its life cycle;
- Continuous monitoring of the device using a risk-based approach; and
- Enabling transparency to users while using real-world evidence post-market.
In response to the discussion paper, a FDA draft guidance titled “Marketing Submission Recommendations for a Predetermined Change Control Plan for Artificial Intelligence/Machine Learning (AI/ML)-Enabled Device Software Functions” was published on April 3, 2023.(14) The draft guidance builds off the general TPLC principles for AI/ML-enabled devices and establishes recommendations and outlines what should be included in a Predetermined Change Control Plan (PCCP) for AI/ML-enabled devices. The PCCP aims to improve the current submission pathway to allow adaptations and improvements that are inevitable with AI/ML-enabled devices, as seen with the subsequent and sequential 510(k) submissions by BrainScope. These PCCP submission recommendations include a Description of Modifications, Modification Protocol, and Impact Assessment to improve efficiency of the medical device pathway when updates to the software post-market are needed. Specifically, the types of software modifications include:
- Retraining the ML algorithm using new data within the same intended population and range of input;
- Expanding the ML algorithm to include new inputs of the same data type, or limited modifications related to new inputs; and
- Adjusting the use and performance of the device to be used for a subset of the original population, and retraining using data specifically for that population subset.
Given the types of changes that the PCCP outlines, we can exemplify BrainScope and show how their 510k submissions could potentially have fit the PCCP criteria. One modification that BrainScope previously implemented was a new input of neurocognitive assessments that resulted in novel data categories which would most likely not qualify for the PCCP, as this modification introduced a new type of data that was not previously incorporated into the original submission. Another modification implemented was a new classification of injury using EEG data. This modification most likely would classify for the PCCP, as it uses the same type of data as presented within the original submission and maintains the original intended use of the device.
The PCCP encourages the developers of these devices to actively consider and plan future software changes and their potential impact on patients. Outlining the potential modifications of the device in the PCCP may help the regulatory pathway become more efficient in the future and support the possible introduction of “unlocked” algorithms into AI/ML-enabled devices.
Regulatory Pathways for AI/ML tools in Drugs and Biologics – Novel Developments
Published on May 5, 2023, the FDA discussion paper “Using Artificial Intelligence & Machine Learning in the Development of Drug and Biological Products” discusses the current use of AI/ML tools in the development of drugs and biologics and considerations for usage in clinical trials.(15) AI/ML is being developed to augment many essential aspects of clinical drug research including recruitment, selection and stratification of trial participants, dose/dosing regimen optimization, adherence, retention, site selection, clinical trial data collection, management and analysis, and clinical endpoint assessment. AI/ML methods are used to predict a participant’s clinical outcome of a drug trial based on their baseline characteristics, such as demographic information, vital signs, labs, medical imaging data, etc. These methods can help identify high-risk participants as well as patients who are more likely to respond to treatment. By using AI/ML-enabled algorithms for clinical endpoint assessments that are necessary for evaluating the safety risks and efficacy of the trials, potential or real-time safety issues can be identified.
Additionally, the FDA’s Innovative Science and Technology Approaches for New Drugs (ISTAND) Pilot Program supports the development of drug development tools (DDT) that do not qualify for existing DDT pathways but may benefit drug development. The ISTAND program is a three-step process that can ultimately lead to the qualification of the DDT.(16) In January 2024, ISTAND accepted a submission for their first AI/ML-based digital health technology for neuroscience that automates the assessment of depression and anxiety symptoms via a ML model to produce clinician-reported outcomes based on the Hamilton Depression Rating Scale (HAM-D) and the Hamilton Anxiety Rating Scale (HAM-A) scores.(17) The FDA will soon provide feedback and guidance on developing a qualification plan, which is the first step of the program.
Through the provision of discussion papers, draft guidances, and the organization of programs dedicated to new developments that use AI/ML, the FDA has demonstrated recognition of AI/ML’s vital role in advancing healthcare. Although the development of AI/ML itself is not new, the intersection with neurology is relatively new, and continues to develop every year.
Pittsburgh Highlight: ML Neurological Developments
With several major universities and a growing tech ecosystem in Pittsburgh, the region is beginning to make an impact with AL/ML-enabled technologies. In 2021, there were 69,473 TBI-related deaths, per the CDC.(18) University of Pittsburgh School of Medicine data scientists Shandong Wu, Ph.D., Matthew Pease, M.D., Dooman Arefan, Ph.D., and University of Pittsburgh Medical Center professor of neurological surgery David Okonkwo, M.D., Ph.D., recognized the clinical need and how the impact of informed decisions for clinicians can affect treatment of TBI patients in critical conditions. Their recognition led to the development of a ML algorithm which uses brain scans and vital signs to determine the risk of death and potential outcome following TBI. Study results showed that the algorithm can accurately predict a patient’s mortality (area under the receiver operating characteristic curve [AUC], 0.92 vs. 0.80; P = <.001) and unfavorable outcomes (AUC, 0.88 vs. 0.82; P = .04) at six months following a traumatic incident.(19, 20) The clinical validation of the algorithm is one of the first steps in developing an AI/ML tool to assist in TBI diagnosis and helping to support future clinical decisions.
CLINICAL RESEARCH STRATEGIES ACKNOWLEDGMENT OF AI/ML
Clinical Research Strategies (CRS) recognizes the importance and relevance of AI/ML in healthcare and clinical research. By staying current on guidelines that define the usage of AI/ML in different clinical areas, we maintain a strong understanding of the developing pathways of AI/ML-based regulatory submissions as well as recognize the role of AI/ML within future clinical trials. This unique know-how enables us to mentor our clients on the best practices and regulatory pathways for their product development life cycle. CRS also encourages the reflection of good clinical practices (GCPs) and human factors testing when developing and assessing AI/ML-enabled technologies and their use within the patient population, especially those who have compromised health conditions such as TBIs.
References
1. Center for Drug Evaluation and Research. (2023, May 16). Artificial Intelligence and Machine Learning (AI/ML) for Drug Development. Food and Drug Administration. https://www.fda.gov/science-research/science-and-research-special-topics/artificial-intelligence-and-machine-learning-aiml-drug-development
2. Hansch, Corwin., & Fujita, Toshio. (1964). p-σ-π Analysis. A Method for the Correlation of Biological Activity and Chemical Structure. Journal of the American Chemical Society, 86(8), 1616–1626. https://doi.org/10.1021/ja01062a035
3. Joshi, G., Jain, A., Shalini Reddy Araveeti, Adhikari, S., Garg, H., & Bhandari, M. (2024). FDA-Approved Artificial Intelligence and Machine Learning (AI/ML)-Enabled Medical Devices: An Updated Landscape. Electronics, 13(3), 498–498. https://doi.org/10.3390/electronics13030498
4. Grand View Research. (2022). Artificial Intelligence Market Size & Trends. AI Industry Report, 2025. Grand View Research. https://www.grandviewresearch.com/industry-analysis/artificial-intelligence-ai-market
5. Center for Devices and Radiological Health. (2021). Artificial Intelligence and Machine Learning (AI/ML)-Enabled Medical Devices. FDA. https://www.fda.gov/medical-devices/software-medical-device-samd/artificial-intelligence-and-machine-learning-aiml-enabled-medical-devices
6. Device Classification Under Section 513(f)(2)(De Novo) – BrainScope Ahead 100. (n.d.). www.accessdata.fda.gov. Retrieved April 9, 2024, from https://www.accessdata.fda.gov/scripts/cdrh/cfdocs/cfpmn/denovo.cfm?id=DEN140025
7. Brainscope. (2024). https://www.brainscope.com/
8. Proposed Regulatory Framework for Modifications to Artificial Intelligence/Machine Learning (AI/ML)-Based Software as a Medical Device (SaMD). (2019). Food and Drug Administration. https://www.fda.gov/files/medical%20devices/published/US-FDA-Artificial-Intelligence-and-Machine-Learning-Discussion-Paper.pdf
9. Dhunnoo, P. (2022, February 22). Locked And Adaptive Algorithms In Healthcare: Differences, Importance And Regulatory Hurdles. The Medical Futurist. https://medicalfuturist.com/locked-and-adaptive-algorithms-in-healthcare-differences-importance-and-regulatory-hurdles/
10. 510(k) Premarket Notification – BrainScope Ahead 200. (n.d.). www.accessdata.fda.gov. Retrieved April 9, 2024, from https://www.accessdata.fda.gov/scripts/cdrh/cfdocs/cfpmn/pmn.cfm?ID=K143643
11. 510(k) Premarket Notification – BrainScope Ahead 300. (n.d.). www.accessdata.fda.gov. Retrieved April 9, 2024, from https://www.accessdata.fda.gov/scripts/cdrh/cfdocs/cfpmn/pmn.cfm?ID=K161068
12. 510(k) Premarket Notification – BrainScope Ahead 400. (n.d.). www.accessdata.fda.gov. Retrieved April 9, 2024, from https://www.accessdata.fda.gov/scripts/cdrh/cfdocs/cfpmn/pmn.cfm?ID=K183241
13. 510(k) Premarket Notification – BrainScope Ahead 500. (n.d.). www.accessdata.fda.gov. Retrieved April 9, 2024, from https://www.accessdata.fda.gov/scripts/cdrh/cfdocs/cfpmn/pmn.cfm?ID=K190815
14. Marketing Submission Recommendations for a Predetermined Change Control Plan for Artificial Intelligence/Machine Learning (AI/ML)-Enabled Device Software Functions Draft Guidance for Industry and Food and Drug Administration Staff DRAFT GUIDANCE. (2023). Food and Drug Administration. https://www.fda.gov/media/166704/download
15. Using Artificial Intelligence & Machine Learning in the Development of Drug & Biological Products: Discussion Paper and Request for Feedback. (2023, May 5). Food and Drug Administration. https://www.fda.gov/media/167973/download
16. Center for Drug Evaluation and Research. (2021, February 10). Innovative Science and Technology Approaches for New Drugs (ISTAND) Pilot Program. Food and Drug Administration. https://www.fda.gov/drugs/drug-development-tool-ddt-qualification-programs/innovative-science-and-technology-approaches-new-drugs-istand-pilot-program
17. Center for Drug Evaluation and Research. (2024). FDA’s ISTAND Pilot Program accepts submission of first artificial intelligence-based and digital health technology for neuroscience. Food and Drug Administration. https://www.fda.gov/drugs/drug-safety-and-availability/fdas-istand-pilot-program-accepts-submission-first-artificial-intelligence-based-and-digital-health
18. CDC. (2019). TBI Data and Statistics. Centers for Disease Control and Prevention. https://www.cdc.gov/TraumaticBrainInjury/data/index.html
19. Machine Learning Model Can Steer Traumatic Brain Injury Patients to Life-Saving Care. (2022, April 26). UPMC. https://www.upmc.com/media/news/042522machine-learning-model
20. Pease, M., Dooman Arefan, Barber, J., Yuh, E. L., Puccio, A. M., Hochberger, K., Nwachuku, E. L., Roy, S., Casillo, S. M., Temkin, N. R., Okonkwo, D. O., Wu, S., Neeraj Badjatia, Bodien, Y. G., Duhaime, A.-C., V. Ramana Feeser, Ferguson, A. R., Foreman, B., Gardner, R. C., & Gopinath, S. P. (2022). Outcome Prediction in Patients with Severe Traumatic Brain Injury Using Deep Learning from Head CT Scans. Radiology, 304(2), 385–394. https://doi.org/10.1148/radiol.212181
ABOUT THE AUTHOR
Anna Carpio
Clinical Research Associate
Anna earned a bachelor’s degree in psychology Cum Laude with Honors in 2023, from the University of Pittsburgh, and established an interest in neuroscience research with brain injury patients. Anna worked for Dr. Anthony Kline at the Safar Research Center, where she studied the effects of pharmaceuticals on experimental traumatic brain injuries. Through this research, she had the opportunity to attend the SACNAS conference as a Travel Scholar and won an Outstanding Research Presentation award.
Ms. Carpio was published as a corresponding author in Experimental Neurology, for her research contributions to “A bridge to recovery: acute amantadine prior to environmental enrichment after brain trauma augments cognitive benefit.” She has experience as a clinical assistant in rehabilitating TBI patients, and as a peer specialist at Western Psychiatric Hospital, furthering her passion for neuroscience and psychology.
ANNA CARPIO
Clinical Research Associate